Orchid's team of genetic experts has developed a genetic risk score (GRS) for atrial fibrillation.
Written by Orchid Team
Orchid has developed advanced genetic risk scores (GRS) for a variety of diseases. Here we present our data on our GRS of atrial fibrillation.
Atrial Fibrillation
Atrial fibrillation is a form of cardiac arrhythmia where the atrium of the heart beats rapidly and irregularly, which increases the risk of strokes by fivefold [1]. The precise cause of the disease is unknown [2]. The disease is substantially influenced by genetic factors, and its heritability is estimated to be approximately 60% based on a study that used data on 120,000 individuals of European ancestry [3]. Treatment of atrial fibrillation depends on a patient’s risk score (CHA2DS2-VASc), which uses patient age and other risk factors to predict risk of stroke, but can include anticoagulation, slowing the heart rate, and more [4].
Genetic risk score (GRS)
A genetic risk score quantifies the degree to which an individual’s genetics increases their likelihood of developing a specific disease. The GRS for atrial fibrillation includes 1,097,774 variants and was developed based on the variants identified in a study that analyzed the genomes of 133,073 individuals of European ancestry. The weights of the model were adjusted for linkage disequilibrium using PRScs software. The study included 17,931 cases (individuals with atrial fibrillation) and 115,142 healthy controls [5].

Clinical Impact and Prevalence
Atrial fibrillation is the most common form of cardiac arrhythmia; about 3-6 million Americans are believed to be living with the disease, and that figure is predicted to rise to 12 million by 2030 (3% of the population) [6]. The main complication of atrial fibrillation is the approximately 5-fold increased risk of stroke. Treatment is aimed at reducing the risk of stroke through slowing the heart rate (“rate control”), anticoagulating the blood, and occasionally catheter ablation [4].
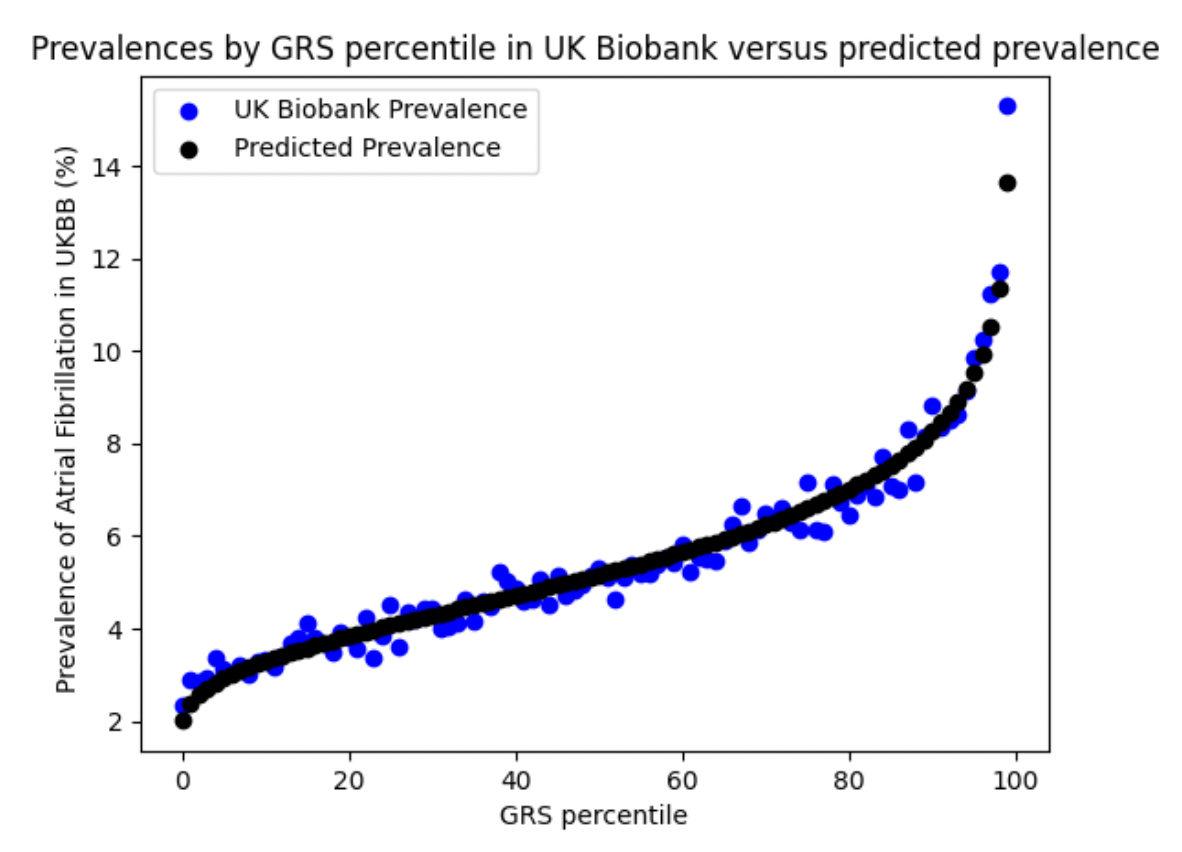
In the UK Biobank, cases were identified using self reported atrial fibrillation (UK Biobank field 20002, code) relevant ICD-10 diagnosis and death codes. See supplementary table for more details. In the validation, prevalence of the disease increased with GRS.
Prevalence at the higher end of the GRS distribution is significantly higher than the average prevalence in the UK Biobank (5.5%).
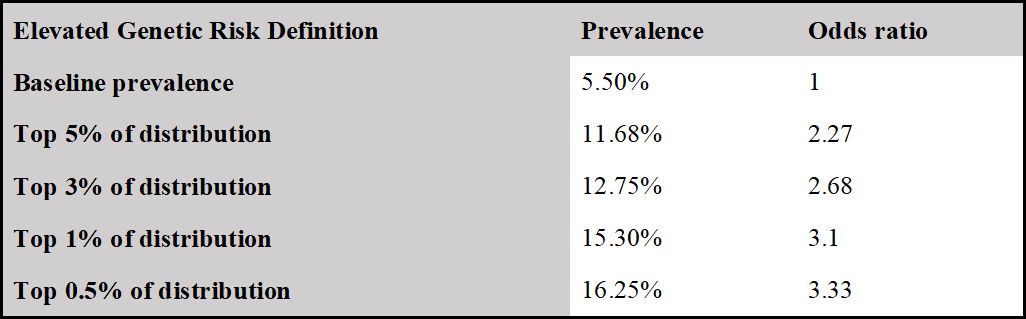
Identification of adults at 2-3 times the baseline risk of atrial fibrillation
Adults in the 99th percentile of genetic risk develop atrial fibrillation at 2.78 times the baseline rate, with an odds ratio of 3.1 Baseline rate is the prevalence of the disease in the entire reference population.
Comparison to Published Benchmarks
Orchid’s model achieves comparable stratification performance with an AUC of 0.750 compared to the benchmark at 0.750.
We compared the performance of our model as validated on the UK Biobank with the performance of the best model in Khera et al. To make a comparison of models, we restricted our validation sample to those in Phase II of the UK Biobank release, as in Khera et. al. In the first column, we give the results for our predictor with the phenotype as described above. In the second, we report the metrics for the best-performing predictor in Khera et. al, using the same validation setup as ours.

1 Khera et al
Citations
1. Wolf PA, Abbott RD, Kannel WB. Atrial fibrillation as an independent risk factor for stroke: the Framingham Study. Stroke. 1991;22: 983–988.
2. NHS. Atrial fibrillation - Causes. [cited 4 Jan 2022]. Available: https://www.nhs.uk/conditions/atrial-fibrillation/causes/
3. Weng L-C, Choi SH, Klarin D, Smith JG, Loh P-R, Chaffin M, et al. Heritability of Atrial Fibrillation. Circ Cardiovasc Genet. 2017;10. doi:10.1161/CIRCGENETICS.117.001838
4. January CT, Wann LS, Calkins H, Chen LY, Cigarroa JE, Cleveland JC Jr, et al. 2019 AHA/ACC/HRS Focused Update of the 2014 AHA/ACC/HRS Guideline for the Management of Patients With Atrial Fibrillation: A Report of the American College of Cardiology/American Heart Association Task Force on Clinical Practice Guidelines and the Heart Rhythm Society in Collaboration With the Society of Thoracic Surgeons. Circulation. 2019;140: e125–e151.
5. Khera AV, Chaffin M, Aragam KG, Haas ME, Roselli C, Choi SH, et al. Genome-wide polygenic scores for common diseases identify individuals with risk equivalent to monogenic mutations. Nat Genet. 2018;50: 1219–1224.
6. CDC. Atrial Fibrillation. 27 Sep 2021 [cited 4 Jan 2022]. Available: https://www.cdc.gov/heartdisease/atrial_fibrillation.htm
Appendix: Disease case identification and number of cases in UK Biobank

*Type 1 diabetes was defined as a combination the following inclusion and exclusion criteria:
- Self-diagnosed diabetes (any type)
- No self-diagnosed Type 2 diabetes
- Age of diabetes onset between 0 and 20 years
- Started insulin within one year of diagnosis of diabetes