Orchid's team of genetic experts has developed a genetic risk score (GRS) for Class III Obesity.
Written by Orchid Team
Orchid has developed advanced genetic risk scores (GRS) for a variety of diseases. Here we present our data on our GRS of Class III Obesity.
Class III Obesity
Class III (morbid) obesity is a condition defined by having a Body Mass Index (BMI) above 40. Obesity increases the risk of cardiovascular disease, diabetes, and other chronic conditions [1], and more severe obesity increases the risk disproportionally. Obesity has become much more common over the last century because of changes in the food environment [2], but within most studied countries, BMI is moderately heritable, with an estimated heritability of 47% to 90% [3].
A genetic risk score quantifies the degree to which an individual’s genetics increases their likelihood of developing a specific disease. The GRS for obesity includes 1,490,767 variants and was developed based on the variants identified in a study that analyzed genomes of 288,016 individuals of European ancestry affected by obesity (Khera et al. 2019).

Clinical Impact and Prevalence
Severe obesity has increased in prevalence since the early 1900’s, and the CDC reported that American rates of severe obesity (BMI >40) reached 9.2% in 2017, with obesity in general at a prevalence of 42% [4]. Obesity is inversely correlated with educational attainment, such that less educated men and women have higher rates of obesity [4]. Overall mortality, as well as disease-specific mortality, increases with higher levels of obesity [5]. Treatment of obesity generally begins with a lower-calorie diet, but several classes of medications, as well as bariatric surgery, are also used [6] when appropriate. Bariatric surgery, which is highly effective but carries some risk, is an option for patients with severe obesity (BMI >40) or BMI > 35 with at least 1 obesity-related disease, such as type 2 diabetes.
Performant severe obesity risk stratification
Validated using a large cohort of real world individuals with obesity
Individuals in the 99th percentile of genetic risk have a 9.6% prevalence of obesity, compared to the baseline rate of 1.87%. Baseline rate is the prevalence of the disease in the entire reference population.
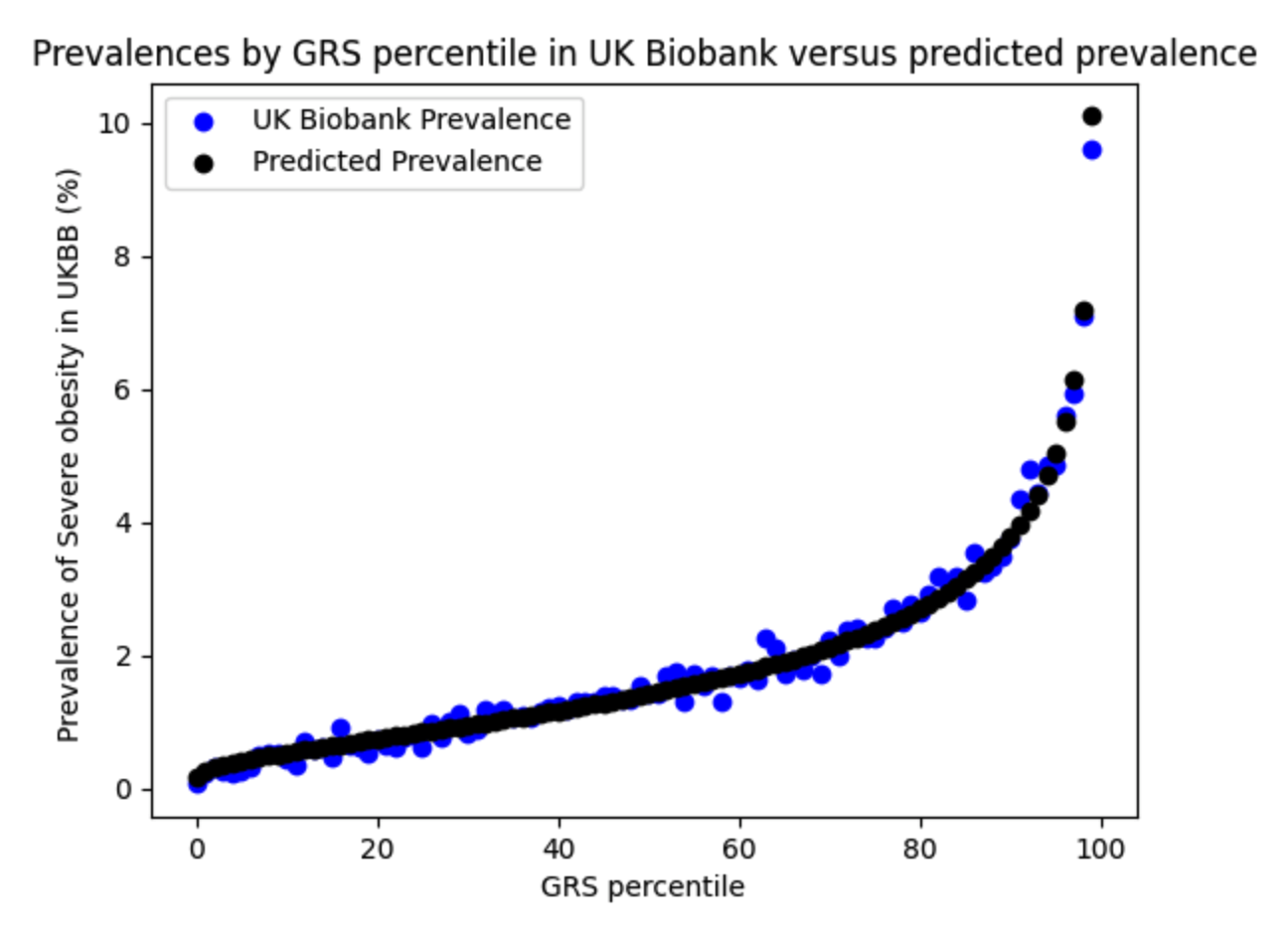
Validation in UK Biobank. In the UK Biobank, cases were identified using measured Class III Obesity (BMI >40). In the validation, prevalence of obesity increased with GRS. We restricted our analysis to self-reported British individuals whose genetic ancestry matched their self-identification.
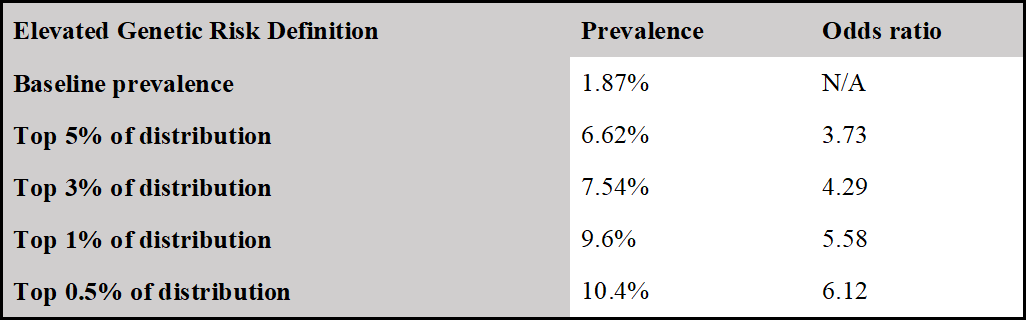
Identification of people at 5-6 times the baseline risk of Class III Obesity
Individuals in the 99th percentile of genetic risk develop Class III Obesity (BMI > 40) at 5.13 times the baseline rate, with an odds ratio of 5.58. Baseline rate is the prevalence of the disease in the entire reference population.
Comparison to Published Benchmarks
Orchid’s model achieves an AUC of 0.724. The paper from which we obtained our GRS predictor (Khera et al. 2019) does not contain an AUC metric, so we cannot compare our predictor with the benchmark.
Citations
1. Hruby A, Manson JE, Qi L, Malik VS, Rimm EB, Sun Q, et al. Determinants and Consequences of Obesity. Am J Public Health. 2016;106: 1656–1662.
2. Bleich S, Cutler D, Murray C, Adams A. Why is the developed world obese? Annu Rev Public Health. 2008;29: 273–295.
3. Elks CE, den Hoed M, Zhao JH, Sharp SJ, Wareham NJ, Loos RJF, et al. Variability in the heritability of body mass index: a systematic review and meta-regression. Front Endocrinol . 2012;3: 29.
4. CDC. Adult Obesity Facts. In: Centers for Disease Control and Prevention [Internet]. 12 Nov 2021 [cited 25 Feb 2022]. Available: https://www.cdc.gov/obesity/php/data-research/adult-obesity-facts.html
5. Prospective Studies Collaboration, Whitlock G, Lewington S, Sherliker P, Clarke R, Emberson J, et al. Body-mass index and cause-specific mortality in 900 000 adults: collaborative analyses of 57 prospective studies. Lancet. 2009;373: 1083–1096.
6. Ruban A, Stoenchev K, Ashrafian H, Teare J. Current treatments for obesity. Clin Med . 2019;19: 205–212.
7. Khera AV, Chaffin M, Wade KH, Zahid S, Brancale J, Xia R, et al. Polygenic Prediction of Weight and Obesity Trajectories from Birth to Adulthood. Cell. 2019;177: 587–596.e9.
8. Faucon, Annika. Improving the computation efficiency of polygenic risk score modeling: Faster in Julia. https://www.biorxiv.org/content/10.1101/2021.12.27.474263v1.full
9. Smoller, Jordan. Polygenic prediction via Bayesian regression and continuous shrinkage priors. https://www.nature.com/articles/s41467-019-09718-5